MA234 大数据导论与实践(二)
Big Data (II)
IV. Classification
本章内容较多,先写下本章主要内容:
本章涉及分类算法,主要会提及 KNN 算法,决策树算法和朴素贝叶斯算法(是分类算法中最基础的几种)
其中,每种算法的应用里涵盖了一些多用概念,如剪枝操作、似然函数计算等。介绍三种基本算法后,本章还涉及模型评估,讲解如何通过不同问题使用不同的算法以得到最优的结果
注:本章含有不亚于数据预处理章节的数学公式,要求理解公式基本内涵。
K-Nearest Neighbor (KNN)
AlgorithmSupervised learning method, especially useful when prior knowledge on the data is very limited.
Low bias, high variance : just for small
k
Advantages : not sensitive to outliers (异常值距离一般较远) , easy to implement and parallelize, good for large training set
Drawbacks : need to tune (调节) $k$, take large storage, computationally intensive (计算缓慢,算力要求高)
- Input : training set $D_{train} = \{(x_1, y_1),\cdots,(x_N, y_N)\}$, a test sample $x$ without label $y$, $k$ and distance metric $d(x, y)$
- Output : predicted label $y_{pred}$ for $x$
- Compute $d(x, x_j)$ for each $(x_j , y_j) \in D_{train}$
- Sort the distances in an ascending order, choose the first $k$ samples $(x_{(1)}, y_{(1)}),\cdots,(x_{(k)} , y_{(k)})$
- Make majority vote $y_{pred} = \text{Mode}(y_{(1)},\cdots, y_{(k)})$
Time Complexity : $O(mndK)$ where $n$ is the number of training samples, $m$ is the number of test samples, $d$ is the dimension, and $K$ is the number of nearest neighbors
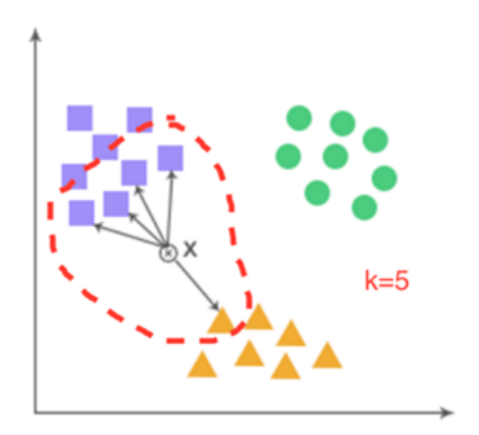
Similarity and Divergence
- Cosine similarity
- Jaccard similarity for sets $A$ and $B$ : $Jaccard(A,B)=\Large{\frac{|A\cap B|}{|A\cup B|}}$
- Kullback-Leibler(KL) divergence : $d_{KL}(P||Q) = E_P log \large{\frac{P(x)}{Q(x)}}$ , measures the distance between two probability distributions $P$ and $Q$ ; in discrete case, $d_{KL}(p||q) = \sum^m_{i=1} p_i log \large{\frac{p_i}{q_i}}$ (CDF of $P$ and $Q$)
Tuning k
- Different
k
value can lead to totally different results. ( model overfit the data whenk = 1
, bad for generalization ) - M-fold Cross-validation (CV) to tune
k
:- partition the dataset into M parts ( M = 5 or 10 ) , let $\kappa : \{1,\cdots, N\} \to \{1,\cdots, M\}$ be randomized partition index map (随机分布索引映射) . The CV estimate of prediction error (预测误差的CV估计) is
$CV(\hat f,k)=\large{\frac{1}{N}} \sum_{n=1}^{N}L(y_i,\hat f^{-\kappa(i)}(x_i,k))$
- partition the dataset into M parts ( M = 5 or 10 ) , let $\kappa : \{1,\cdots, N\} \to \{1,\cdots, M\}$ be randomized partition index map (随机分布索引映射) . The CV estimate of prediction error (预测误差的CV估计) is
k’s value | $k=1$ (complex model) | $k=\infty$ (simplier model) |
---|---|---|
Bias | decrease | increase |
Variance | increase | $0$ |
Generalization | overfitting (train-set friendly) | underfitting (test-set friendly) |
Bayes Classifier (Oracle Classifier)
- Assume $Y \in \mathcal{Y} = \{1, 2, . . . , C\}$, the classifier $f : \mathcal X → \mathcal Y$ is a piecewise (分段) constant function
- For 0-1 loss $L(y, f )$, the learning problem is to minimize
- Bayes rule : $f^{∗} (x) = \arg \max_c P(Y = c|X = x)$ , “the most probable label under the conditional probability on x”
- Bayes Error Rate (贝叶斯误差) : $\text{inf}_{f}\varepsilon (f)=$ $\color{red}\mathcal E(f^{\ast})$ $=1-P(Y=f^{\ast}(X))$
- Bayes Decision Boundary (贝叶斯决策边界) : the boundary separating the K partition domains in $\mathcal X$ on each of which $f^{ ∗ }(x) \in Y$ is constant. For binary classification, it is the level set on which $P(Y=1|X=x)=P(Y=0|X=x)=0.5$
- Recall : Decision boundary of 15NN is smoother than that of 1NN
- 1NN error rate is twice the Bayes error rate
- Bayes error $=1-p_{c^\ast}(x)$ where $c^\ast=\arg\max_{c}p_c(x)$
- Assume the samples are i.i.d. (独立同分布) , for any test sample $x$ and small $\delta$, there is always a training sample $z \in B(x, \delta)$ (the label of $x$ is the same as that of $z$), then 1NN error is
$
\begin{align}
\epsilon=\sum_{c=1}^{C}p_c(x)(1-p_c(z))\overset{\delta\to 0}{\longrightarrow}&1-\sum_{c=1}^{C}p_{c}^{2}(x) \\
\le\ &1-p_{c^\ast}^{2}(x) \\
\le\ &2(1-p_{c^\ast}(x))
\end{align}
$
- Remark : In fact, $\color{green}\epsilon\le 2(1-p_{c^\ast}^{2}(x))-\frac{C}{C-1}(1-p_{c^\ast}^{2}(x))^2$
- We have to consider its radius, texture (质地) , perimeter, area, smoothness, etc. (n-dimension)
- Data scaling : 0-1 scaling or z-score scaling
- Use code to assist
1 | from sklearn.neighbors import KNeighborsClassifier |
Decision Tree
- Tree structure : internal nodes indicate features, while leaf nodes represent classes.
- Start from root, choose a suitable feature $x_i$ and its split point $c_i$ at each internal node, split the node to two child nodes depending on whether $x_i \le c_i$ , until the child nodes are pure.
- Equivalent to rectangular partition of the region.
![]() |
![]() |
---|---|
Rectangular partition |
- How to choose features and split points ?
- Impurity : choose the feature and split point so that after each slit the impurity should decrease the most
- Impurity(M0)-Impurity(M12) > Impurity(M0)-Impurity(M34), choose A as split node ; otherwise choose B
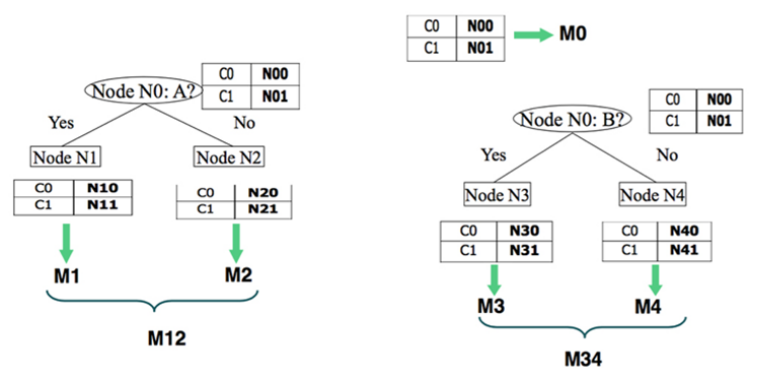
- Impurity Measures
- GINI Index
- Gini index of node $t$ : $Gini(t)=1-\sum_{c=1}^C (p(c|t))^2$ where $p(c|t)$ is the proportion of class-c data in node $t$
- Gini index of a split : $Gini_{split}=\sum_{k=1}^{K}\frac{n_k}{n}Gini(k)$ where $n_k$ is the number of samples in the child node $k$, $n=\sum_{k=1}^{K} n_k$
- Choose the split so that $Gini(t) − Gini_{split}$ is maximized
- Information Gain
- Entropy at $t$ : $H(t) = −\sum_{c=1}^{C}p(c|t)\log_2 p(c|t)$ ,
- where $t$ is the node and $\color{blue}c$ represents that this node is chosen.
- Maximum at $log_2 C$, when $p(c|t)=\frac{1}{C}$
- Minimum at $0$, when $p(c|t)=1$ for some $c$
- Misclassification Error
- Misclassification error at t : $\text{Error}(t) = 1 − \max_c p(c|t)$ (use majority vote)
- Maximum at $1−\frac{1}{C}$, when $p(c|t) = \frac{1}{C}$
- Minimum at $0$, when $p(c|t)=1$ for some $c$
- GINI Index
- Compare Three Measure
- Gini index and information gain should be used when growing the tree
- In pruning, all three can be used (typically misclassification error)
Algorithm | Type | Impurity Measure | Child Nodes | Target Type |
---|---|---|---|---|
ID3 | Discrete | Info Gain | $k\ge 2$ | Discrete |
C4.5 | Discrete, Continuous | Info Gain | $k\ge 2$ | Discrete |
C5.0 | Discrete, Continuous | Info Gain | $k\ge 2$ | Discrete |
CART | Discrete, Continuous | Gini Index | $k=2$ | Discrete, Continuous |
- Tree Pruning (剪枝)
- Too complex tree structure easily leads to overfitting (分类太细,模型太复杂)
- Prepruning : set threshold (阈值) $\delta$ for impurity decrease (剔除杂质) in splitting a node ; if $\Delta \text{Impurity}_{split} \gt \delta$, do slitting, otherwise stop
- Postpruning : based on cost function (provided $|T|$ and $\alpha$)
- $\color{red}\text{Cost}_{ \alpha}(T)=\sum_{t=1}^{|T|}n_t\ \text{Impurity}(t)+\alpha|T|$
- Input: a complete tree $T$, $\alpha$
- Output: postpruning tree $\text{T}_{\alpha}$
- Compute $\text{Impurity}(t)$ for $\forall t$
- Iteratively merge child nodes bottom-up : Suppose $\text{T}_{A}$ and $\text{T}_{B}$ are the trees before and after merging, do merging if $\text{Cost}_{ \alpha}(\text{T}_{A}) \ge \text{Cost}_{ \alpha}(\text{T}_{B})$ (剪枝前损失更大)
- Pros and Cons
- Advantage
- Easy to interpret and visualize : widely used in finance, medical health, biology, etc.
- Easy to deal with missing values (treat as new data type)
- Could be extended to regression
- Disadvantage
- Easy to be trapped at local minimum because of greedy algorithm (贪心)
- Simple decision boundary : parallel lines to the axes (Recall Pic above)
- Advantage
Naive Bayes (朴素贝叶斯)
- Based on Bayes Theorem and conditional independency assumption on features (Recall Bayes Classifier)
- Bayes Theorem : $\Large{P(Y|X)=\frac{P(X|Y)P(Y)}{P(X)}}$
- $P(Y)$ is prior prob. distribution (先验概率分布) , $P(X|Y )$ is likelihood function (似然函数) , $P(X)$ is evidence (边际概率) , $P(Y |X)$ is posterior prob. distribution (后验概率分布).
- The core problem of machine learning is to estimate $P(Y |X)$
- Let $X = \{X_1, . . . , X_d \}$, for fixed sample $X = x$, $P(X = x)$ is independent of $Y$ , by Bayes Theorem, $P(Y|X=x)\propto P(X=x|Y)P(Y)$
- Assume conditional independency of $X_1, \cdots, X_d$ given $Y = c$ : $P(X=x|Y=c)=\prod_{i=1}^{d}P(X_i=x_i|Y=c)$
- Naive Bayes Model :
$
\color{red}\hat y =\arg \max_c P(Y=c)\prod_{i=1}^{d}P(X_i=x_i|Y=c)
$
Maximum Likelihood Estimate (MLE)
- Estimate $P(Y = c)$ and $P(X_i = x_i |Y = c)$ from the dataset $D = \{(\textbf{x}_1, y_1), \cdots ,(\textbf{x}_n, y_n)\}$
- MLE for $P(Y = c)$ : $P(Y = c) =\Large{\frac{ \sum_{i=1}^{n} I(y_i=c)}{n}}$
- When $X_i$ is discrete variable with range $\{v_1, \cdots , v_K\}$, MLE for $P(X_i = v_k |Y = c) =\Large{\frac{ \sum_{i=1}^{n} I(x_i = v_k |y_i = c)}{ \sum_{i=1}^{n} I(y_i = c)}}$
( if $X_i$ is continuous, just do discretization on it and use this formula )
Model Assessment
Confusion Matrix
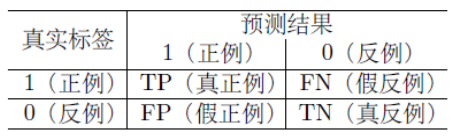
- Representation
- T & F : represents truth of label (标签是否真实)
- P & N : represents aspect of label (标签的两面)
Two-class classification:
- $\text{Accuracy} =\large{\frac{\text{TP+TN}}{\text{TN+FN+FP+TP}}}$, not a good index when samples are imbalanced
- $\text{Precision}=\large{\frac{\text{TP}}{\text{TP+FP}}}$
- TPR : $\text{Recall} = \large{\frac{\text{TP}}{\text{TP+FN}}}$ ; important in medical diagnosis (回收)
- F score : $F_{\beta} = \large{\frac{(1+\beta^2)\text{Precision}\times\text{Recall}}{\beta^2 \times \text{Precision}+\text{Recall}}}$ , e.g. $F_1$ score for $\beta=1$
- FPR : $\text{Specifity} = \large{\frac{\text{TN}}{\text{TN+FP}}}$ ; recall for negative samples
Receiver Operating Characteristic (ROC, 受试者工作特征) and Area Under ROC (AUC)
- Aim to solve class distribution imbalance problem
- Set different threshold (阈值) $t$ for continuous predicted values.
- Compute TPR vs. FPR for all $t$ and plot ROC curve
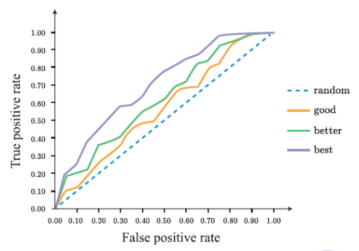
- Beware: Don’t view the “curve” as a function, but as a continuous set of points.
- Higher ROC implies better performance (How to measure ? AUC)
- AUC: compute the area under ROC curve. The larger the better. Model is good for test set if $AUC \gt 0.75$
Cohen’s Kappa Coefficient
Since ROC and AUC is complex to be quantified, we need a
coe
to indicate it.We use an example to explain how to quantified it.
$
\begin{align}
&\text{Cohen’s Kappa Coefficient: }& &\kappa=\frac{p_o-p_e}{1-p_e}=1-\frac{1-p_o}{1-p_e} \\
&& &p_e=\sum_{c=1}^{C}\frac{n_c^{pred}}{N}\frac{n_c^{true}}{N}
\end{align}
$
- $p_o$ is the accuracy
- $p_e$ is the hypothetical probability of chance agreement
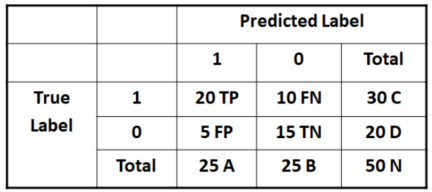
E.g. $\large{p_o=\frac{20+15}{50}=0.7}$, $\large{p_e=\frac{25}{50}\times\frac{20}{50}+\frac{25}{50}=0.5}$, then $\large{\kappa=0.4}$
- $\kappa \in [-1,1]$, $\kappa\ge 0.75$ for good performance and $\kappa\lt 0.4$ for bad one.
V. Regression
Linear Model
Linear model :
- For Univariate linear model, $y = w_0 + w_1x + \epsilon$, where $w_0$ and $w_1$ are regression coefficients, $\epsilon$ is the error or noise
Assume $\epsilon ∼ \mathcal N (0, \sigma^2)$, where $σ^2$ is a fixed but unknown variance; then $y|x ∼ \mathcal N (w_0 + w_1x, σ^2)$
which means $L(\hat w_0,\hat w_1)$ is minimized (残差最小).
- For multivariate linear model, $y=f(\textbf{x})=w_0+w_1x_1+w_2x_2+\cdots+w_px_p + \epsilon$
- where $w_0, w_1,\cdots, w_p$ are regression coefficients, $\textbf{x} = (x_1,\cdots, x_p)^T$ is the input vector whose components are independent variables or attribute values, $\epsilon \thicksim \mathcal N(0, σ^2)$ is the noise.
- For the size n samples $\{(\textbf{x}_i, y_i)\}$, let $\textbf{y} = (y_1, \cdots , y_n)^T$ be the response or dependent variables, $\textbf{w} = (w_0, w_1, \cdots, w_p)^T$, we construct a matrix $\textbf{X}=[\textbf{1}_n, (\textbf{x}_1, \cdots,\textbf{x}_n)^T]\in \mathbb R^{n \times(p+1)}$ , and $\textbf{\varepsilon}=(\epsilon_1,\cdots,\epsilon_n)^T \thicksim \mathcal N(\textbf{0},\sigma^2\textbf{l}_n)$
$
\begin{align}
&\textbf{y}=\textbf{X}\textbf{w} + \varepsilon\\ \\
&\textbf{X}=
\begin{pmatrix}
1 & x_{11} & \cdots & x_{1p} \\
1 & x_{21} & \cdots & x_{2p} \\
\vdots & \vdots & \ddots & \vdots \\
1 & x_{n1} & \cdots & x_{np}
\end{pmatrix}
\end{align}
$
Least Square (LS) 最小二乘法
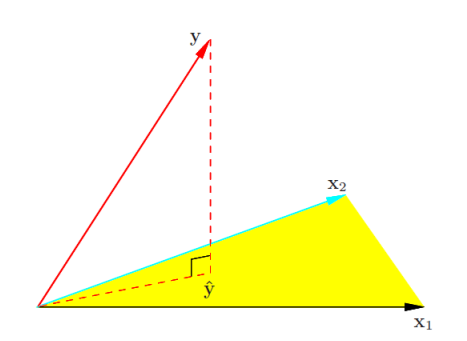
- From geometry aspect, we should minimize the residual sum-of-square (残差平方和):
$\text{RSS}(\textbf{w})=\sum_{i=1}^{n} (y_i-w_0-w_1x_1-\cdots-w_px_p)^2=|\textbf{y} - \textbf{X} \textbf{w}|_{2}^2$- When $\textbf{X}^T\textbf{X}$ is invertible, the minimizer $\hat{\textbf{w}}$ satisfy : (可证明 $\hat w$ 是无偏估计)
$
\nabla_{\textbf{w}}\text{RSS}(\hat{\textbf{w}})=0 \Rightarrow \hat{\textbf{w}}=(\textbf{X}^T \textbf{X})^{-1}\textbf{X}^T \textbf{y}
$
- Then prediction $\hat{\textbf{y}}=\textbf{X}(\textbf{X}^T \textbf{X})^{-1}\textbf{X}^T \textbf{y}= \textbf{P} \textbf{y}$ is a projection of $\textbf{y}$ onto the linear space spanned by the column vectors of $\textbf{X}$; (As Pic 15 show)
- $\textbf{P}=\textbf{X}(\textbf{X}^T \textbf{X})^{-1}\textbf{X}^T$ is the projection matrix satisfying $\textbf{P}^2 = \textbf{P}$ (Recall: Linear Algebra)
- Then prediction $\hat{\textbf{y}}=\textbf{X}(\textbf{X}^T \textbf{X})^{-1}\textbf{X}^T \textbf{y}= \textbf{P} \textbf{y}$ is a projection of $\textbf{y}$ onto the linear space spanned by the column vectors of $\textbf{X}$; (As Pic 15 show)
Optimal Method: Ordinary least square (OLS)
- Get mean values from sample set: $\bar y=\frac{1}{n}\sum_{i=1}^{n}y_i$ , $\bar{\textbf{x}}=\frac{1}{n}\sum_{i=1}^{n}{ \textbf{x}_i}$
- Centralize data (minus by $\bar y$ and $\bar{\textbf{x}}$) and calculate $RSS(\tilde{\textbf{w}})$
- Prediction $\hat{\textbf{y}}= \textbf{P} \textbf{y}$ is the projection (投影) of $\textbf{y}$ on the linear space spanned by the columns of $\textbf{X}$.
$\mathcal X= \text{Span} \{ \textbf{x}_{\cdot ,0}, \textbf{x}_{\cdot ,1},\cdots, \textbf{x}_{\cdot ,p}\}$ , recall that $ \textbf{x}_{\cdot ,0}= \textbf{1}_n$- If $\{ \textbf{x}_{\cdot ,0}, \textbf{x}_{\cdot ,1},\cdots, \textbf{x}_{\cdot ,p}\}$ forms a set of orthonormal basis (标准正交基) , then $\hat{\textbf{y}}=\sum_{i=0}^{p}<\textbf{y}, \textbf{x}_{\cdot ,i}> \textbf{x}_{\cdot ,i}$
- If not, do orthogonalization by Gram-Schmidt procedure for the set $\{ \textbf{x}_{\cdot ,0}, \textbf{x}_{\cdot ,1},\cdots, \textbf{x}_{\cdot ,p}\}$
- From mathemetic aspect, it’s about MLE (Result the same)
- Likelihood function: $L((\textbf{w},\textbf{X}),\textbf{y})=P(\textbf{y}|(\textbf{X}, \textbf{w}))=\prod_{i=1}^{n}P(y_i|(\textbf{x}_i, \textbf{w}))$
- Find MLE: $\hat{\textbf{w}}=\arg \max_{\textbf{w}} L(\textbf{w} ; \textbf{X}, \textbf{y})$ (E.g. For $P(y_i|(\textbf{x}_i, \textbf{w}))=\frac{1}{\sqrt{2\pi}\sigma} \Large{e^{-\frac{(y_i-w_0-w_1x_{i1}-\cdots-w_px_{ip})^2}{2\sigma^{2}}}}$)
- (2.) is equivalent to its log-function:
E.g. $l(\textbf{w} ; \textbf{X}, \textbf{y})= \log{L(\textbf{w} ; \textbf{X}, \textbf{y})}=-n\log(\sqrt{2\pi}\sigma)-\frac{1}{2\sigma^{2}} \sum_{i=1}^{n} (y_i-w_0-w_1x_{i1}-\cdots-w_px_{ip})^2$ - Then get the same minimizer as LS : $\hat{\textbf{w}}=(\textbf{X}^T \textbf{X})^{-1}\textbf{X}^T \textbf{y}$
Shortcomings of Fitting Nonlinear Data (上述方法仅适合线性回归)
- Evaluating the model by Coefficient of Determination $R^2$
- $R^2 := 1-\frac{ \text{SS}_{res}}{ \text{SS}_{tot}}$ ($=\frac{ \text{SS}_{reg}}{ \text{SS}_{tot}}$ only for linear regression), where
- $ \text{SS}_{tot} = \sum_{i=1}^{n} (y_i-\bar y)^2$ is the total sum of squares
- $ \text{SS}_{reg} = \sum_{i=1}^{n} (\hat y_i-\bar y)^2$ is the regression sum of squares
- $ \text{SS}_{res} = \sum_{i=1}^{n} (y_i-\hat y_i)^2$ is the residual sum of squares.
- The larger the $R^2$, the better the model !
- $R^2 := 1-\frac{ \text{SS}_{res}}{ \text{SS}_{tot}}$ ($=\frac{ \text{SS}_{reg}}{ \text{SS}_{tot}}$ only for linear regression), where
- Multicolinearity [多重共线性]
- If the columns of $\textbf{X}$ are almost linearly dependent (multicolinearity), then $\det(\textbf{X}^{T}\textbf{X})\approx 0$, the diagonal entries in $(\textbf{X}^{T}\textbf{X})^{-1}$ is quite large, leading to a large variances of $\hat{\textbf{w}}$ (inaccurate).
- Remedies (补救措施): ridge regression (岭回归), principal component regression (主属性回归), partial least squares regression (部分最小二乘回归), etc.
- Overfitting
- Linear regression easily to be overfitted when introducing more variables.
- Solution: Regularization
Bias-Variance Decomposition
- Bias (偏差): $\text{Bias}(\hat f(\textbf{x}))=\text{E}_\text{train}\hat f(\textbf{x})-f(\textbf{x})$ , average accuracy of prediction for the model (deviation from the truth)
- Variance (方差): $\text{Var}(\hat f(\textbf{x}))=\text{E}_\text{train}(\hat f(\textbf{x})-\text{E}_\text{train}\hat f(\textbf{x}))^2$ , variability of the model prediction due to different data set (stability)
$
\color{red}
\text{E}_\text{train}\text{R}_\text{exp}(\hat f(\textbf{x}))=\text{E}_\text{train}\text{E}_\text{P}[(y-\hat f(\textbf{x}))^2|\textbf{x}] = \underbrace{\text{Var}(\hat f(\textbf{x}))}_{\text{variance}}+\underbrace{\text{Bias}^2(\hat f(\textbf{x}))}_{\text{bias}}+\underbrace{\sigma^2}_{\text{noise}}
$
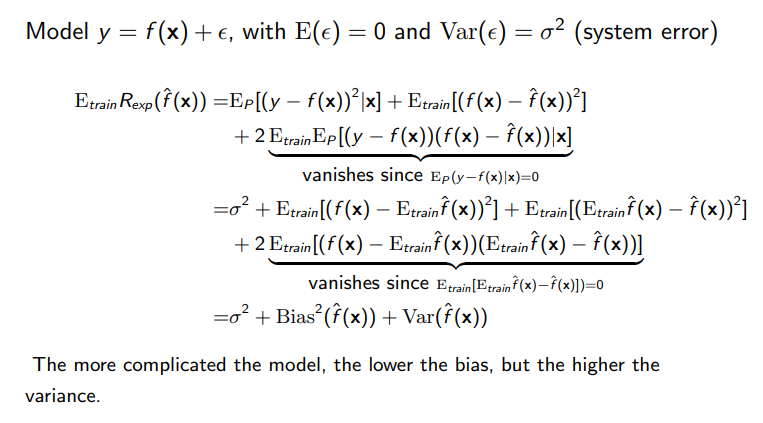
- The more complicated the model, the lower the bias, but the higher the variance.
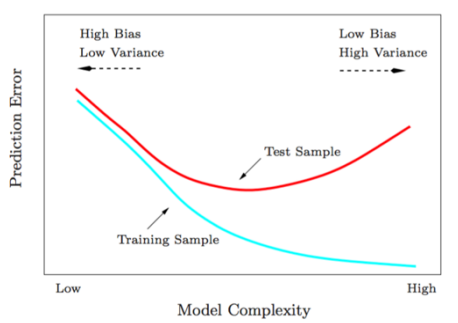
- kNN Regression
- kNN can be used to do regression if the mode (majority vote) is replaced by mean : $\hat f(x)=\frac{1}{k} \sum_{ x_{(i)} \in N_{k}(x)} y_{(i)}$
- Generalization error of kNN regression is
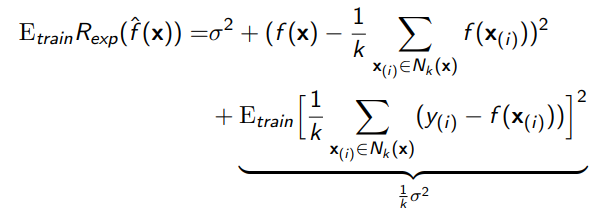
where we have used the fact that $E_{ \text{train}} y_{i} = f(\textbf{x}_{i})$ and $\text{Var}(y_i)=\sigma^2$
- For small $k$, overfitting, bias ↓, variance ↑
- For large $k$, underfitting, bias ↑, variance ↓
Regularization (正则化)
Why we need Regularization ?
- In high dimensions, the more the input attributes, the larger the variance
- Shrinking some coefficients or setting them to zero can reduce the overfitting
- Using less input variables also help interpretation with the most important variables
- Subset selectionµretaining only a subset of the variables, while eliminating the rest variables from the model
Best-Subset Selection
- find for each $k ∈ \{0, 1, \cdots , p\}$ the subset $S_k \subset \{1,\cdots, p\}$ of size $k$ that gives the smallest $\text{RSS}(\textbf{w}) = \sum_{i=1}^n (y_i − w_0 − \sum_{j\in S_k} w_j x_{ij})^2$
- Noted that the best subset of size $k + 1$ may not include the the variables in the best subset of size $k$
- Choose $k$ based on bias-variance tradeoff, usually by AIC and BIC(贝叶斯信息量), or practically by cross-validation
Forward-stepwise selection
- Start with the intercept (截距?) $\bar y$ , then sequentially add into the model the variables that improve the fit most (reduce RSS most)
- QR factorization helps search the candidate variables to add
- Greedy algorithm : the solution could be sub-optimal
Backward-stepwise selection
- Start with the full model, then sequentially delete from the model the variables that has the least impact on the fit most
- The candidate for dropping is the variable with the smallest Z-score
- Can only be used when $n > p$ in order to fit the full model by OLS
Regularization by Penalties
- Add a penalty term, in general $l_q$ - norm
$
\sum_{i=1}^{n}(y_i-w_0-w_1x_1-\cdots-w_px_p)^2+\lambda |\textbf{w}|^q_q=|\textbf{y}-\textbf{X}\textbf{w}|^2+\lambda |\textbf{w}|^q_q
$
- By arranging $\lambda$ , we can correct the overfitting (bias inc. & var dec.)
q = 2
for Ridge Regression &q = 1
for LASSO Regression
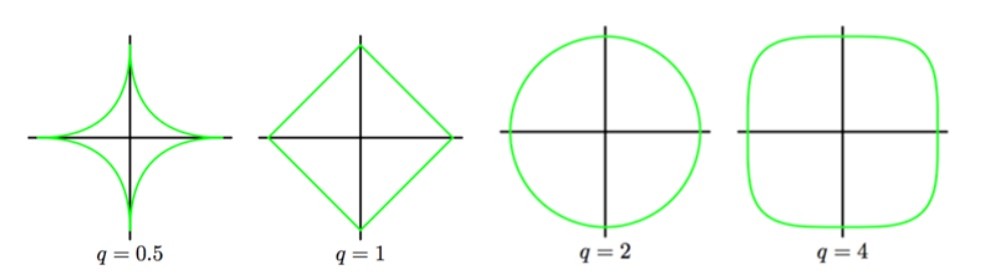
Ridge Regression
$\hat w=\arg \underset{w}\min {\|y-Xw\|_2^2}+\lambda\|w\|_2^2$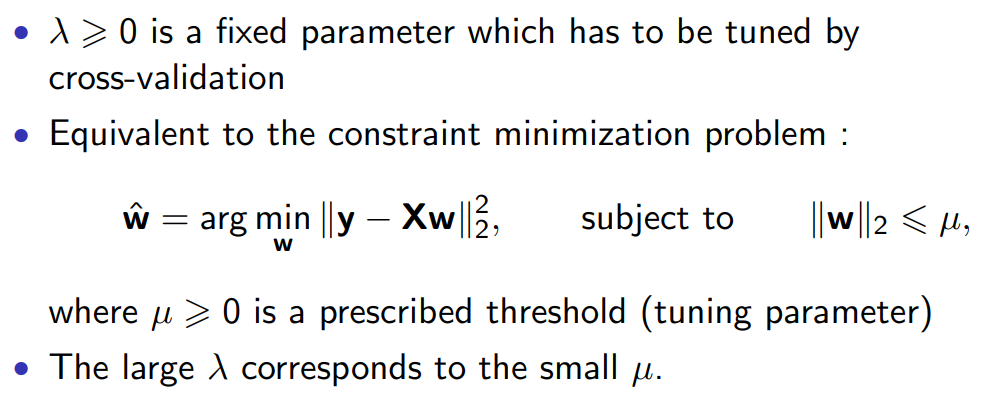
Solving Ridge Regression
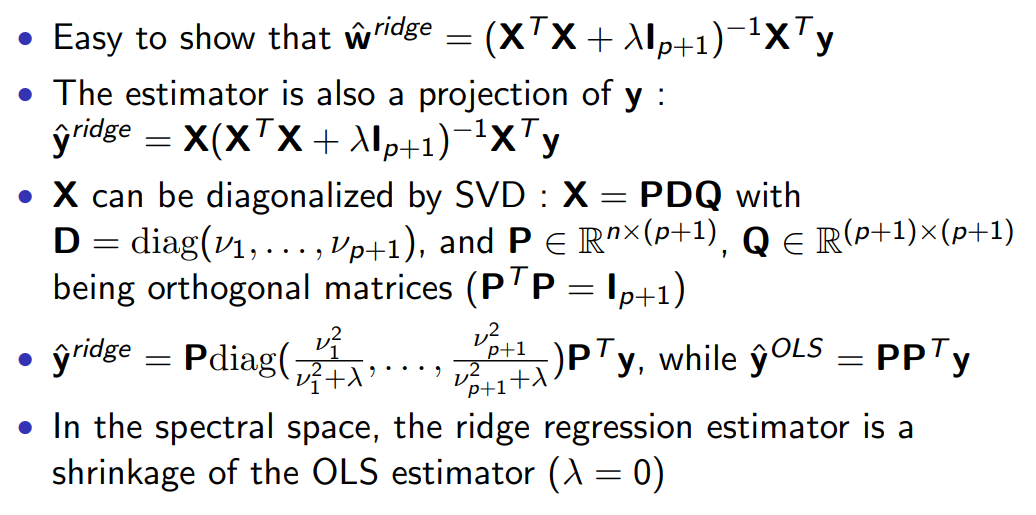
Bayesian Viewpoint of Ridge Regression
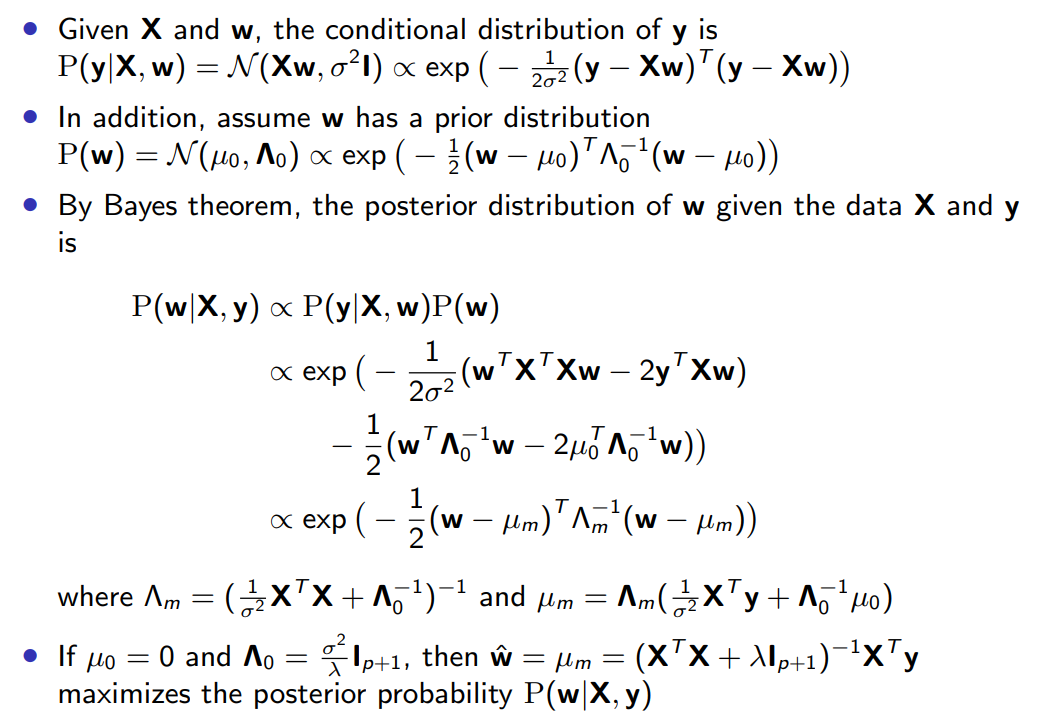
LASSO Regression
$\hat w=\arg \underset{w}\min {\|y-Xw\|_2^2}+\lambda\|w\|_1$Can be used to estimate the coefficients and select the important variables simultaneously
Reduce the model complexity, avoid overfitting, and improve the generalization ability
Two Rpoperties :
- Shrinkage (将所有点收缩)
- Selection (将近点归零,远点收缩)
![]() |
![]() |
$
\hat w_i^{\text{lasso}} = (|\hat w^{OLS}_i| − \lambda)+\text{sign}(\hat w^{OLS}_i)
$
- Solving LASSO by LARS (最小角回归算法)
- Start with all coefficients $w_i$ equal to zero
- Find the predictor $x_i$ most correlated with $y$ (一般认为夹角最小的即是)
- Increase the coefficient $w_i$ in the direction of the sign of its correlation with $y$. Take residuals $r = y − \hat y$ along the way. Stop when some other predictor $x_k$ has as much correlation with $r$ as $x_i$ has (调整参数 $w$ 直至下一个分量夹角最小)
- Increase $(w_i, w_k)$ in their joint least squares direction, until some other predictor $x_m$ has as much correlation with the residual $r$
- Continue until all predictors are in the model
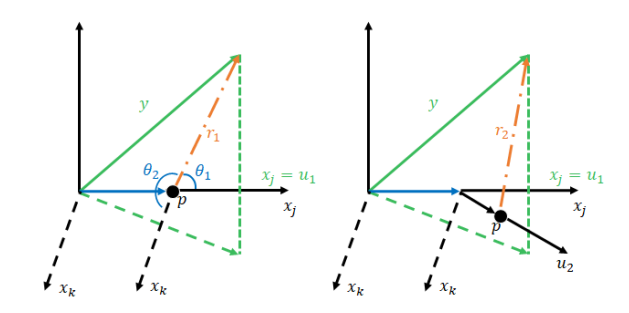
Optional: Maximum A Posteriori (MAP) Estimation
Given $\theta$ , the conditional distribution of $\textbf{y}$ is $P(\textbf{y}|\theta)$
MAP choose the point of maximal posterior probability :
$\hat{\theta}^{MAP}=\arg \underset{\theta}\max{(\log P(\textbf{y}|\theta)+\log P(\theta))}$
If $\theta=\textbf{w}$, and we choose the log-prior [对数先验] (i.e. normal prior $\mathcal N(0, \frac{\sigma^2}{\lambda} \textbf{I})$ ) , we revocer the ridge regression.
Different log-prior lead to different penalties (Not general case. Some penalties may not be the logarithms[对数] of probability distributions, some other penalties depend on the data)
Related Regularization Models
- Elastic net (混合回归) : $\hat{\textbf{w}}=\arg\min_w|y-Xw|_2^2+\lambda_1|\textbf{w}|^2_2+\lambda_2|\textbf{w}|_1$
- Group LASSO (对不同分组进行回归) : $\hat{\textbf{w}}=\arg\min_w|y-Xw|_2^2+\sum_{g=1}^{G}\lambda_{g}|\textbf{w}_{g}|_2$ , where $\textbf{w}=(w_1,\cdots,w_G)$ is the group partition of $\textbf{w}$.
- Dantzig Selector : …
- Smoothly clipped absolute deviation (SCAD) penalty
- Adaptive LASSO
ADMM Used in LASSO Problem
Altinating Direction Method of Multipliers (ADMM)
- ADMM [交替方向乘子法] often used to solve problems with two optimized variables which only has equality constraint.
Normal Form as below :
where $x\in R^{n}$ and $z\in R^{m}$ are optimized variables, and in the equality constraint, $A\in R^{p\times n}$ , $B\in R^{p\times m}$ , $c\in R^{p}$ , and $f$ and $g$ are convex functions (凸函数)
- Define Augmented Lagrangian (增广拉格朗日函数)
- If we let $w=\frac{u}{\rho}$ , then we can get simplified form of Augmented Lagrangian
- Algorithm : fixed other variables and update only one of them (Here $\rho\gt 0$ is a penalty parameter)
- Consider LASSO Problem
- To find $\min_{w} \frac{1}{2}|y-Xw|^2_2+\lambda|w|_1$
- Let $w=\beta$ (the constraint : $w-\beta=0$) and rewrite the Augmented Lagrangian : $L_{\rho}(w,\beta,u)=\frac{1}{2}|y-Xw|^2_2+\lambda|\beta|_1+u^T(w-\beta)+\frac{\rho}{2}|w-\beta|_2^2$
Model Assessment
- Mean absolute error (MAE) : $MAE =\frac{1}{n} \sum_{i=1}^{n} |y_i - \hat y_i|$
- Mean square error (MSE) : $MSE =\frac{1}{n} \sum_{i=1}^{n} (y_i - \hat y_i)^2$
- Root mean square error (RMSE) : $RMSE = \sqrt{\frac{1}{n} (y_i - \hat y_i)^2}$
Coefficient of Determination [决定系数] (Recall) : $R^2:=1-\frac{\text{SS}_{\text{res}}}{\text{SS}_{\text{tot}}}$ ,
where $\text{SS}_{\text{tot}}=\sum_{i=1}^n (y_i-\bar y_i)^2$ and $\text{SS}_{\text{res}}=\sum_{i=1}^n (y_i-\hat y_i)^2$- Normally $R^2\in[0,1]$ , but it can be negative (a wrong model making residual too large).
- The larger the $R^2$ , the better the model.
Adjusted Coefficient of Determination
- $n$ is the number of samples, $p$ is the dimensionality (or the number of attributes)
- The larger the $\text{R}_{\text{adj}}^2$ value, the better performance the model
- When adding important variables into the model, $\text{R}_{\text{adj}}^2$ gets larger and $\text{SS}_{\text{res}}$ is reduced
VI. Classification II
Why talk about Regression first ?
- Naive Bayes uses Probability and Mathemetic methods, which is the core of Regression
- Regression all apply MLE, which is connected with Bayes rules.
Logistic Regression
Recall: Linear Regression逻辑回归是一种分类方法(不是回归)
- $E(y|x)=P(y=1|x)=w_0+w_1x$ , but $w_0+w_1x$ may be not probability
- Use Sigmoid function to map it to $\left[0,1\right]$ : $g(z)=\frac{1}{1+e^{-z}}$ , where $z=w_0+w_1x_1+\cdots+w_dx_d$
- Equivalently, $\log{\frac{P(y=1|x)}{1-P(y=1|x)}}=w_0+w_1x_1+\cdots+w_dx_d$
MLE for Logistic Regression
- The prob. distribution for two-class logistic regression model is
- $Pr(y=1|X=x)=\frac{\exp(\textbf{w}^T \textbf{x})}{1+\exp(\textbf{w}^T \textbf{x})}$
- $Pr(y=0|X=x)=\frac{1}{1+\exp(\textbf{w}^T \textbf{x})}$
- Let $P(y=k|X=x)=p_k(\textbf{x};\textbf{w})$, $k=0,1$. The likelihood function is $L(\textbf{w})=\prod_{i=1}^{n} p_{y_i}(\textbf{x}_i;\textbf{w})$
- MLE of $\textbf{w}$ : $\hat{\textbf{w}}=\arg \underset{\textbf{w}}\max L(\textbf{w})$
- Solve $\color{red}\nabla_{\textbf{w}}\log L(\textbf{w})=0$ by Newton-Raphson method
用 MLE 计算 $\hat{\textbf{w}}$ ,需要提前知道 $x$ 的分布,所以逻辑回归是一种分类算法。
Linear Discriminant Analysis (LDA)
Recall: Naive Bayes线性判别分析,是一种监督学习的降维方法(无监督学习一般用PCA,主成分分析来降维)
- By Bayes Theorem: $P(Y|X=x)\propto f_k(\textbf{x})\pi_{k}$ , where $f_k(\textbf{x})=P(\textbf{X}=\textbf{x}|Y=k)$ is be the density function of samples in each class $Y=k$, $\pi_k=P(Y=k)$ is the prior probability.
- Assume $f_k (\textbf{x})$ is multivariate Gaussian (多元高斯分布) : $f_k(x)=\large{\frac{1}{(2\pi)^{p/2} |\Sigma_k}^{1/2}|e^{\frac{1}{2}(x-\mu_k)^T\Sigma_k^{-1}(x-\mu_k)}}$ , with a common covariance matrix (协方差矩阵) $\Sigma_k$ (注:多元高斯可以表示为向量和矩阵乘积的形式,如上)
- For the decision boundary between class $k$ and $l$, the log-ratio of their posteriors (后验) $P(Y|X)$ is
$
\log{\frac{P(Y=k|\textbf{X}=\textbf{x})}{P(Y=l|\textbf{X}=\textbf{x})}}=\log{\frac{\pi_k}{\pi_l}}-\frac{1}{2}(\mu_k+\mu_l)^T\Sigma_k^{-1}(\mu_k-\mu_l)+\textbf{x}^T \Sigma^{-1}(\mu_k-\mu_l)
$
From log-ratio, we can get Linear discriminant functions(e.g. for class $k$) : $\delta_k(\textbf{x})=\textbf{x}^T\Sigma^{-1}\mu_k-\frac{1}{2}\mu_k^T\Sigma^{-1}\mu_k+\log\pi_k$
Then the log-ratio become : $\log{\frac{P(Y=k|\textbf{X}=\textbf{x})}{P(Y=l|\textbf{X}=\textbf{x})}}=\delta_k(\textbf{x})-\delta_l(\textbf{x})$
相减结果是一个一次方程(线性)
Decision Rule(分类依据) : $k^{\ast}=\arg\max_k \delta_k(\textbf{x})$
Two-class LDA
- LDA rule classifies to class 2 if
$
(\textbf{x}-\frac{\hat\mu_1+\hat\mu_2}{2})^T \Sigma^{-1}(\hat\mu_2-\hat\mu_1)+\log{\frac{\hat\pi_2}{\hat\pi_1}}\gt 0
$
- Discriminant direction : $\beta=\Sigma^{-1}(\hat\mu_2-\hat\mu_1)$
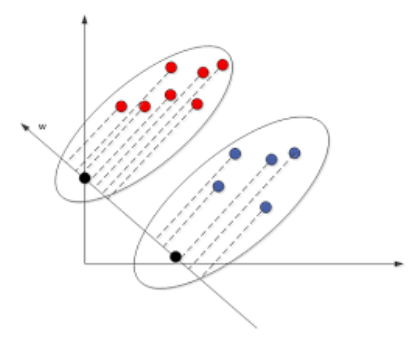
$\hat\mu$ 看作图中椭圆的中心,图中的 $w$ 为投影方向。由上述公式计算可得到样本在投影基向量上的方向,从而判断其类别
从定性上看,投影的作用是降维,选择的投影空间应当是能将不同类数据点在映射后尽可能分开(或同类的点尽可能紧凑)。
Neural Network
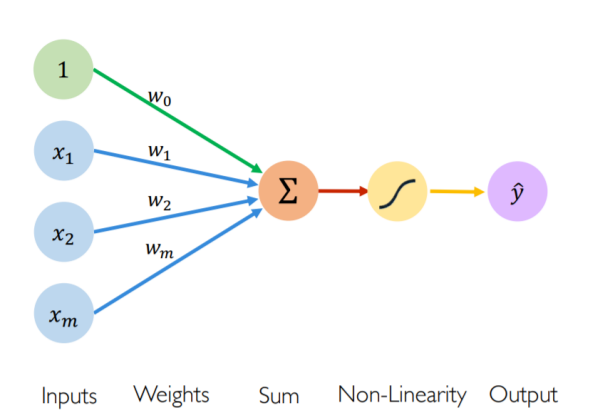
- $\hat y$ is Output
- $g$ is a Non-linear activation function (非线性激活函数)
- $w_0$ is the Bias
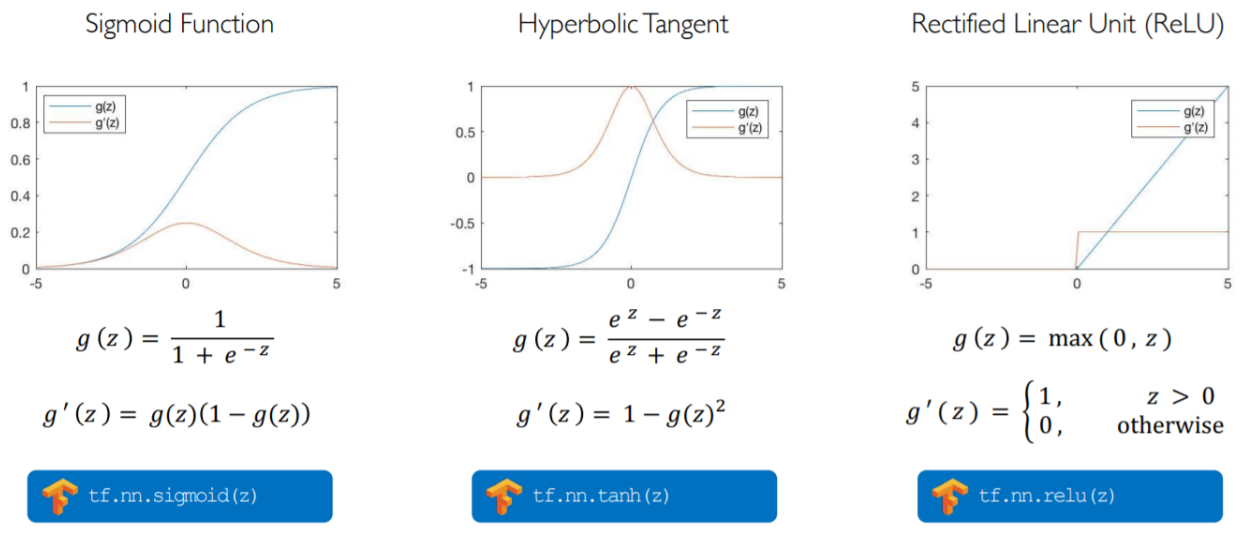
- Single Hidden Layer Neural Network
- $z_i=w_{0,i}^{(1)}+\sum_{j=1}^{m}x_jw_{j,i}^{(1)}$
- $\hat y_i=w_{0,i}^{(2)}+\sum_{j=1}^{d_1}g(z_j)w_{j,i}^{(2)}$
- $x_i\to z_k\to y_j$ , where $z_k$ is the hidden layer
- Hidden Layer can be multiple
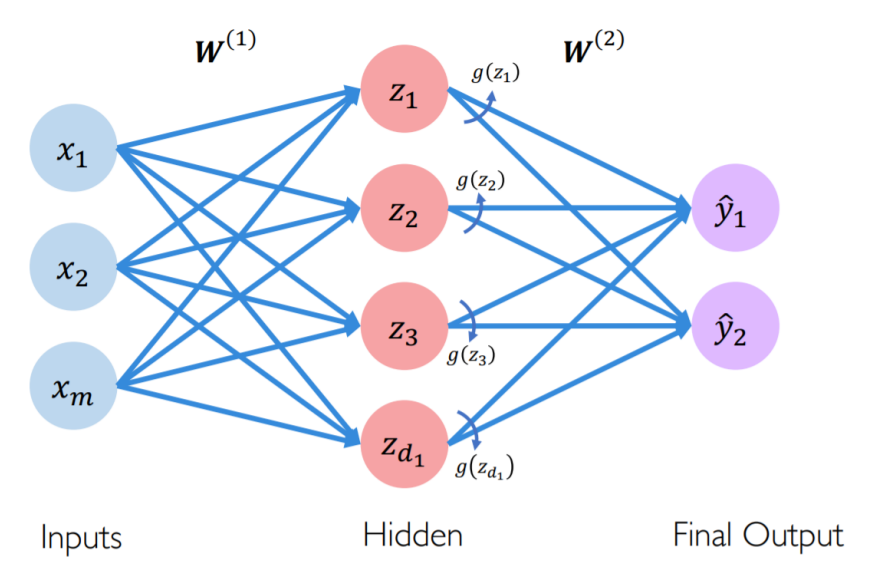
- Thm: Universal Approximation Theorem —— Any function can be approximated by a three-layer neural network within sufficiently high accuracy.
- Why not effective ?
- The width of each layer may be too much (Large calculation !!)
- Now we’re trying to replace width with depth and find the same Theorem (即增加层数,减少每层的神经元)
Loss Optimization
Find $\textbf{W}=\{w^{(0)},w^{(1)},…,w^{(n)}\}$ with lowest loss function
$
\textbf{W}^{\ast}=\underset{\textbf{W}} {\arg\min} \frac{1}{n}\sum_{i=1}^{n}L(f(x^{(i)};\textbf{W}),y^{(i)})=\underset{\textbf{W}} {\arg\min}\ C(\textbf{W})
$
- But for most cases, we should calculate gradient to find $\textbf{W}^{\ast}$
- Use gradient decent to solve: $\frac{\partial{C}}{\partial{\textbf{W}}}$
How to calculate ? (More detail)
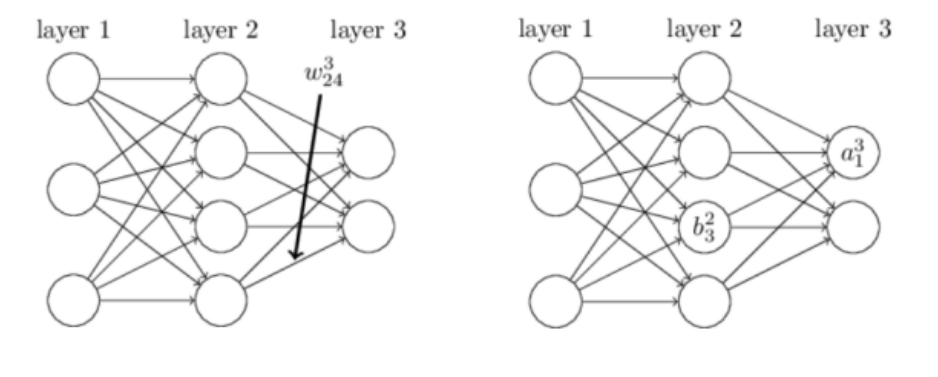
- $w_{jk}^{l}$ is the weight for the connection from the $k^{th}$ neuron in the $(l − 1)^{th}$ layer to the $j^{th}$ neuron in the $l^{th}$ layer.
- More briefly, $b_{j}^{l}=w_{j0}^l$ is the bias of the $j^{th}$ neuron in the $l^{th}$ layer.
$a^l_j$ for the activation of the $j^{th}$ neuron in the $l^{th}$ layer $z_j^l$ : $a^l_j=g(z^l_j)=g(\sum_k w_{jk}^{l}a_k^{l-1} + b_j^l)$
We have define $C(\textbf{W})=\frac{1}{n}\sum_{i=1}^{n}L(f(x^{(i)};\textbf{W}),y^{(i)})$
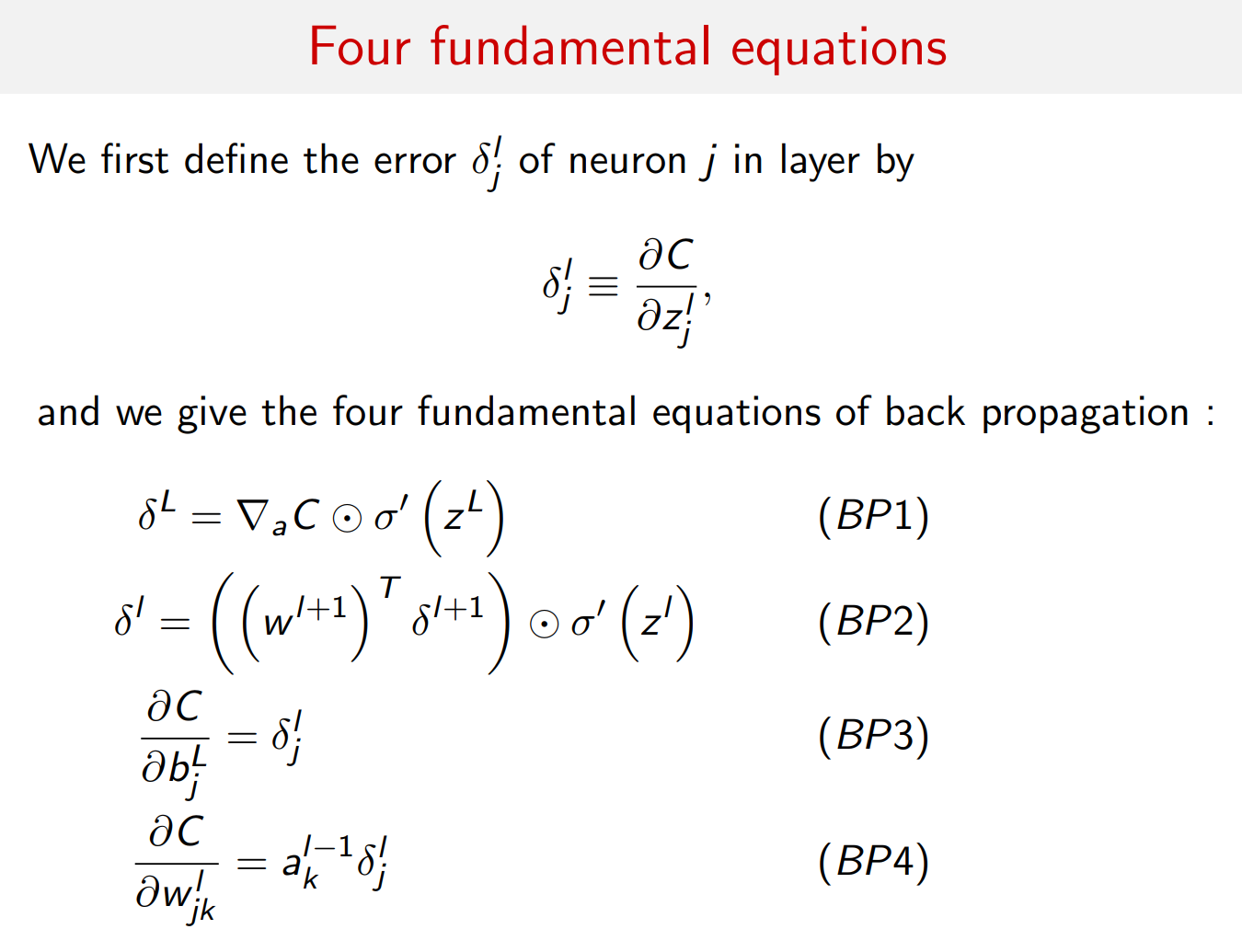
Proof (暂略)
Gradient Descent
Algorithm :
- Initialize weights randomly $\thicksim\mathcal N(0, \sigma^{2})$
- Loop until convergence :
- Pick single data point $i$
- Compute gradient $\frac{\partial J_i(\textbf{W})}{\partial \textbf{W}}$
- Update weights, $\textbf{W} \leftarrow (\textbf{W}-\eta \frac{\partial J(\textbf{W})}{\partial \textbf{W}})$
- Return weights
- Mini-batches lead to fast training ! (need not to calculate all gradient for trainset $x$)
- Can parallelize computation + achieve significant speed increases on GPUs.
Support Vector Machine (SVM)
About SVM
- Use hyperplane [超平面] to separate data : maximize margin
- Can deal with low-dimensional data that are not linearly separated by using kernel functions
- Decision boundary only depends on some samples (support vectors)
How to train
- Training data: $\{(\textbf{x}_1,y_1),(\textbf{x}_2,y_2),…,(\textbf{x}_n, y_n) \}, y_i\in \{-1, 1\}$
- Hyperplane: $S=\textbf{w}^T\textbf{x} + b$ ; Decision function: $f(\textbf{x})=\text{sign}(\textbf{w}^T\textbf{x} + b)$
- Geometric margin between a point and hyperplane : $\large{r_i=\frac{y_i(\textbf{w}^T\textbf{x} + b)}{|\textbf{w}|_2}}$
- Margin between dataset and hyperplane : $\underset{i}\min r_i$
- Maximize margin : $\underset{\textbf{w}, b}\max \underset{i}\min r_i$
Optimization
- Without loss of generality, let $\underset{i}\min y_i(\textbf{w}^T\textbf{x} + b)=1$
- Maximize margin is equivalent to $\underset{\textbf{w}, b}\max \frac{1}{|\textbf{w}|_2}$ , $s.t.\ y_i(\textbf{w}^T\textbf{x} + b)\ge 1,\ i=1,…,n$
- Further reduce to $\underset{\textbf{w}, b} \min \frac{1}{2}|\textbf{w}|_2^2$ , $s.t.\ y_i(\textbf{w}^T\textbf{x} + b)\ge 1,\ i=1,…,n$
- This is primal problem : quadratical programming with linear constraints, computational complexity is $O(p^3)$ where $p$ is dimension
But we use Dual problem optimization(对偶问题优化) most.
- When slater condition is satisfied, $\min \max ⇔ \max \min$
- Dual problem : $\underset{\alpha}\max \underset{\textbf{w}, b}\min L(\textbf{w},b,\alpha)$ —— $L$ is Lagrange function(拉格朗日函数)
Solve for inner minimization problem :
- $\nabla_{\textbf{w}}L=0 \Longrightarrow \textbf{w}^\ast=\sum_i \alpha_iy_i \textbf{x}_i$
- $\frac{\partial L}{\partial b}=0 \Longrightarrow \sum_i\alpha_iy_i=0$
Plug into $L$: $L(\textbf{w}^\ast,b^\ast,\alpha)=\sum_i\alpha_i-\frac{1}{2}\sum_i\sum_j\alpha_i\alpha_jy_iy_j(\textbf{x}_i^T \textbf{x}_j)$
- Dual Optimization:
$
\begin{align}
&\min_\alpha\frac{1}{2}\sum_i\sum_j\alpha_i\alpha_jy_iy_j(\textbf{x}_i^T \textbf{x}_j)-\sum_i\alpha_i, \\
&\text{s.t. }\alpha_i\ge0,\ i=1,…,n,\ \sum_i\alpha_iy_i=0
\end{align}
$
KKT Condition
- Three more conditions from the equivalence of primal and minimax problems
$
\left\{ \begin{array}{l}
\alpha_i^{\ast}\ge 0\\
y_i((\textbf{w}^{\ast})^T \textbf{x}_i+b^{\ast})-1 \ge 0\\
\alpha_i^{\ast}[y_i((\textbf{w}^{\ast})^T \textbf{x}_i+b^{\ast})-1]=0
\end{array}\right.
$
- These together with two zero derivative conditions form KKT conditions
- $\alpha_i^{\ast}\gt 0 \Rightarrow y_i((\textbf{w}^{\ast})^T \textbf{x}_i+b^{\ast})=1$
- Index set of support vectors : $S=\{i|\ \alpha_i \gt 0\}$
- $b=y_s-\textbf{w}^T\textbf{x}_s=y_s-\sum_{i\in S}\alpha_i y_i \textbf{x}^T_i\textbf{x}_s$
- More stable solution :
$
\color{red} b=\frac{1}{|S|}\sum_{s\in S}\left(y_s-\sum_{i\in S}\alpha_i y_i \textbf{x}^T_i\textbf{x}_s\right)
$
Soft Margin
- When data are not linear separable, introduce slack variables (tolerance control of fault) $\xi_i \gt 0$
- Relax constraint to $y_i(\textbf{w}^T\textbf{x} + b) \ge 1-\xi_i$
- Primal problem :
$
\begin{align}
&\underset{\textbf{w}, b} \min \frac{1}{2}|\textbf{w}|_2^2+C\sum_{i=1}^{n}\xi_i\\
&\text{s.t. }y_i(\textbf{w}^T\textbf{x} + b)\ge 1-\xi_i,\ \xi_i \ge 0,\ i=1,…,n
\end{align}
$
- Similar derivation to dual problem : (Difference: add the error coe $C$ as a bound)
$
\begin{align}
&\min_{\alpha}\frac{1}{2}\sum_i \sum_j \alpha_i \alpha_j y_i y_j (\textbf{x}_i^T \textbf{x}_j)-\sum_i \alpha_i \\
&\text{s.t. }0\le \alpha_i \le C,\ i=1,…,n,\ \sum_i\alpha_iy_i=0
\end{align}
$
Nonlinear SVM
- Nonlinear decision boundary could be mapped to linear boundary in high-dimensional space
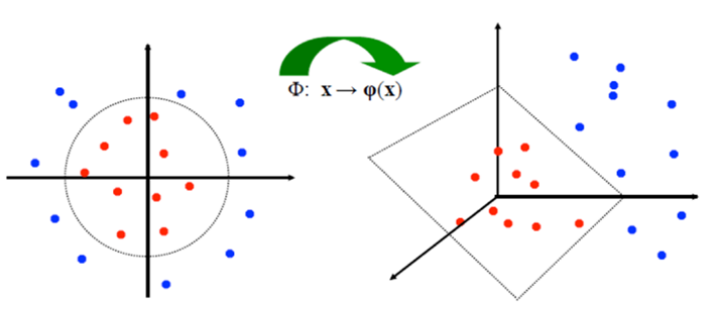
- Modify objective function in dual problem : $\color{red}\frac{1}{2}\sum_i \sum_j \alpha_i \alpha_j y_i y_j (\phi(\textbf{x}_i)^T \phi(\textbf{x}_j))-\sum_i \alpha_i$
- Kernel function as inner product : $K(\textbf{x}_i, \textbf{x}_j)=\phi(\textbf{x}_i)^T \phi(\textbf{x}_j)$
- Q: How to choose Kernel Functions ? A: Arbitrary
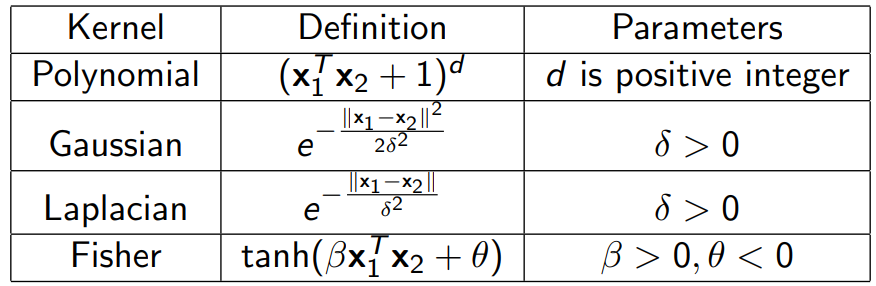